We are living through the fastest technological transition in modern enterprise history. AI is no longer something we’re preparing for. It is already here. The problem is that most enterprise systems, architectures, and governance models were never built to accommodate this kind of velocity.
Leaders now face a question that cuts to the core of strategy and risk: When AI is moving faster than your IT department, what exactly should you do?
How Fast Is AI Moving?

2025 Timeline of Large Language Models by Alan D. Thompson
How Fast Does Enterprise IT Move?
Enterprise IT is built for stability. A Salesforce or SAP deployment can take 12 to 18 months. Every step: procurement; integration; testing; training, governance, is methodical. That model has served us well for decades.
Until now.
Can a CIO Keep Up?
Only if they change how they lead. Because this is not a speed issue. It’s a control issue. It’s a mindset issue. It’s the realization that the traditional tradeoffs between stability and progress are no longer sustainable.
1. Stability Is Not What It Used to Be
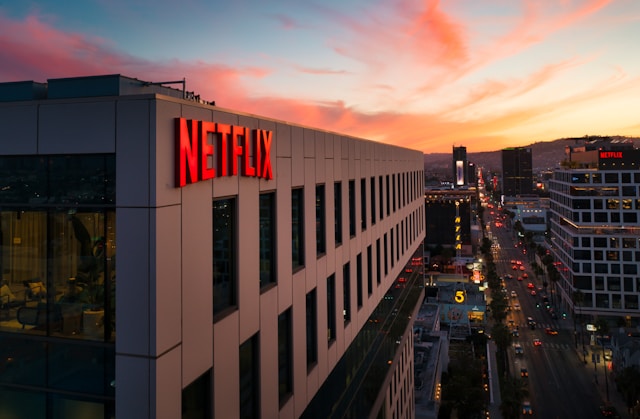
Netflix began its cloud migration in 2009 when AWS was still evolving. Instead of waiting for the infrastructure to mature, they built the reliability they needed themselves—tools like Chaos Monkey to test failure, telemetry for observability, and automated rollback to ensure resilience. They didn’t follow cloud readiness. They defined it.
Enterprise software has always been judged on reliability. You measure it in SLAs, uptime, rollback safety, compliance. These are table stakes.
Today’s AI tools are not built with those expectations in mind. They are powerful, but volatile. They do not ship with user provisioning or change logs. They are updated in production, sometimes without notice.
We cannot apply the same benchmarks we used for ERP systems to tools that reinvent themselves every month.
That doesn’t mean we wait. It means we rethink what stability looks like. We design for adaptability instead.
2. Shadow AI Is Already in the Building
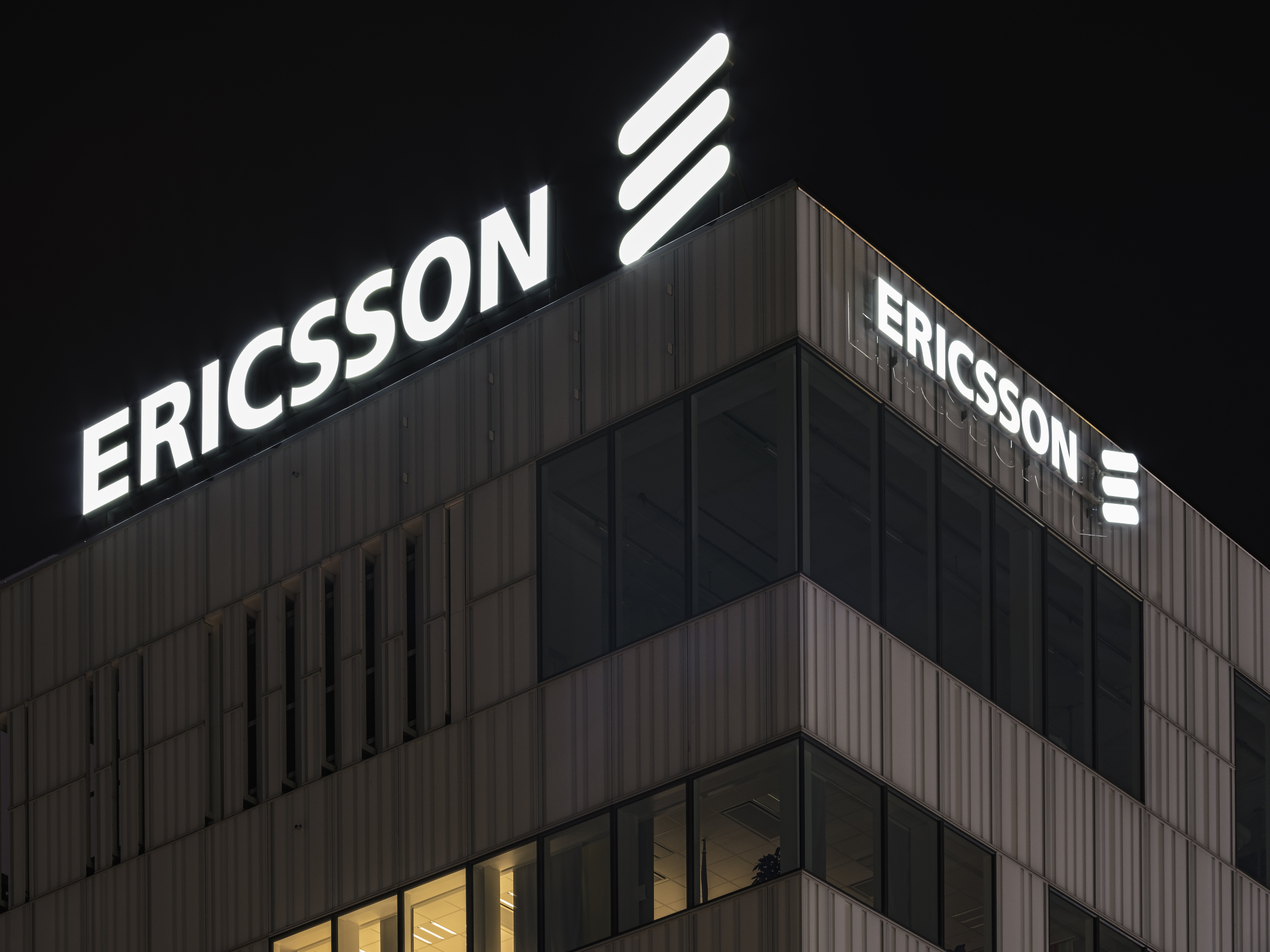
European telecom, Ericcson, launched an internal GenAI assistant with clear usage guidelines and logging. It was rolled out in weeks. Adoption was immediate. And IT had the oversight it needed to protect the business.
Even in companies that have taken no official steps toward AI, adoption is already happening. A marketing team rewrites campaign copy over a weekend using ChatGPT. A developer fixes a bug with GitHub Copilot before anyone in IT even sees the ticket.
An analyst summarizes an entire quarterly report in 30 seconds using a personal device. This is not hypothetical. This is normal. And pretending it is not happening is not a strategy.
Formalizing AI usage is not about slowing people down. It is about bringing visibility and safety to what they’re already doing. It is about giving them tools they can trust — and tools the organization can trust.
3. Use Case Gravity: Some Teams Should Move Now, Others Should Prepare
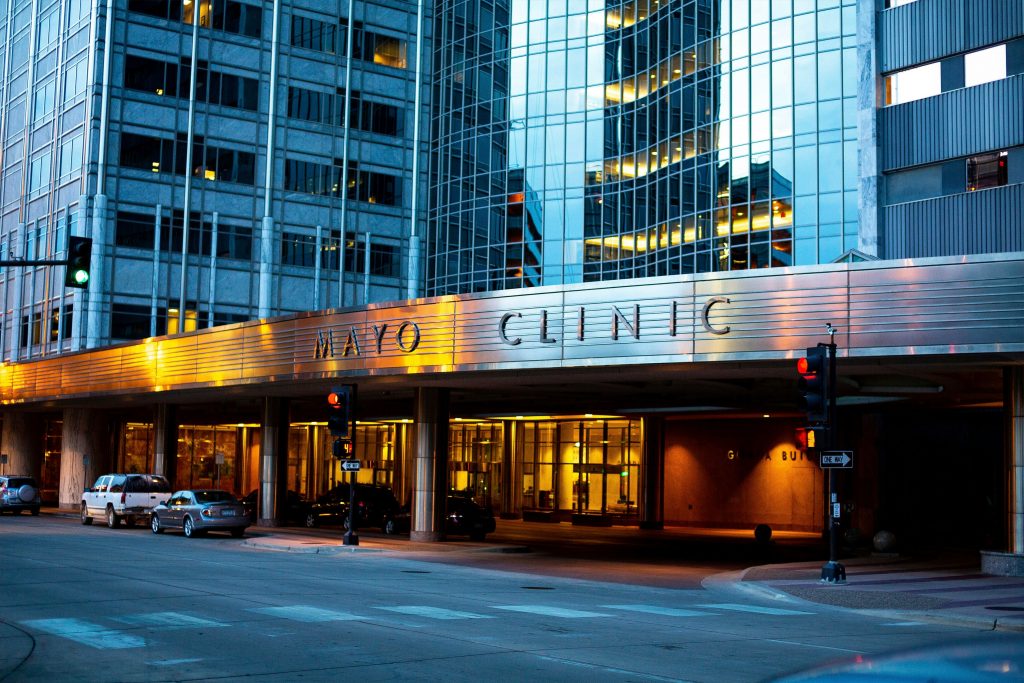
The Mayo Clinic developed an AI system to generate discharge summaries. While the early results were promising, the system occasionally invented facts not present in patient records—a critical failure in a clinical environment. To move forward, they redesigned the approach using a method they called “reverse RAG,” where every AI-generated sentence had to be explicitly traceable back to a verified data point in the record. The lesson was clear: in high-stakes domains, explainability is not optional. It is the price of entry.
AI is not one-size-fits-all. It is not about who adopts first. It is about who adopts with intention.
Some use cases are clearly ready. Merchandising, marketing, customer service — these are high-volume, low-risk environments where speed matters more than perfection.
This is not about risk avoidance. It is about risk alignment. Move fast where you can. Hold back where you must.
4. What Enterprise-Grade AI Actually Requires
Enterprise systems are not just about features. They are about assurance.
If you’ve built or implemented enterprise applications, you already know this. Large organizations are not buying software. They are buying certainty. They are buying the promise that this system will keep the lights on, even under pressure. These applications are the workhorses of the enterprise.
- They must deliver predictable performance, day in and day out.
- They must scale across business units, time zones, and data volumes.
- They must protect sensitive data at every layer.
- And they must evolve without disrupting the thousands of people who rely on them every day to do their jobs.
They need to tolerate faults. They need to be resilient under attack. They need to enforce policy by design and recover quickly when something goes wrong. Most of all, they need to show that the processes they automate are aligned with the business outcomes they are meant to serve.
That is what assurance looks like in the enterprise. And that is what the current generation of AI tools has not yet earned.
5. The Agentic Stack: Where We Are and Where We’re Not
Layer | Component | Ready for Enterprise? | Notes |
Interface | Chat and voice UI, NLU | Yes | Tools like Azure Bot Service and OpenAI are mature. |
Agent Layer | Orchestration, collaboration | Emerging | LangChain and Microsoft Copilot Studio are promising but early. |
Workflow Layer | Triggered execution, memory management | Emerging | Most still require custom vector DBs or Redis for context. |
Integration Layer | APIs to enterprise systems | Yes | Well-supported via REST, GraphQL, and iPaaS tools. |
Data Layer | Context and retrieval | Partial | Retrieval is strong; long-term agent memory is still fragile. |
Governance & Security | Identity, access control, compliance | Partial | Basic controls exist; agent-level policy enforcement is weak. |
Observability & Feedback | Logging, monitoring, feedback loops | Limited | Agent-level monitoring is still immature. |
Reliability & Recovery | Fault tolerance, rollback, multi-agent safety | Missing | No widely adopted solution today for enterprise-grade rollback. |
What This Means for Leaders
Some teams should build now. Because the payoff is immediate and the risks are known.
Others should hold — not because they’re afraid, but because the technology has not yet earned their trust. That is not hesitation. That is leadership.
Either way, you must lead deliberately. Choose where to move. Choose where to wait. But do not drift.
If your organization is ready to define its agentic strategy, I can help.
I work with leaders to assess readiness, identify where Data and AI deliver real advantage, and shape a roadmap that aligns with how your business actually runs. We will not start with tools. We’ll start with outcomes. And we build from there.
Let’s make it work for your people, your process, and your future.